The Future of Food Quality Control: AI's Significant Impact
Recent Post:
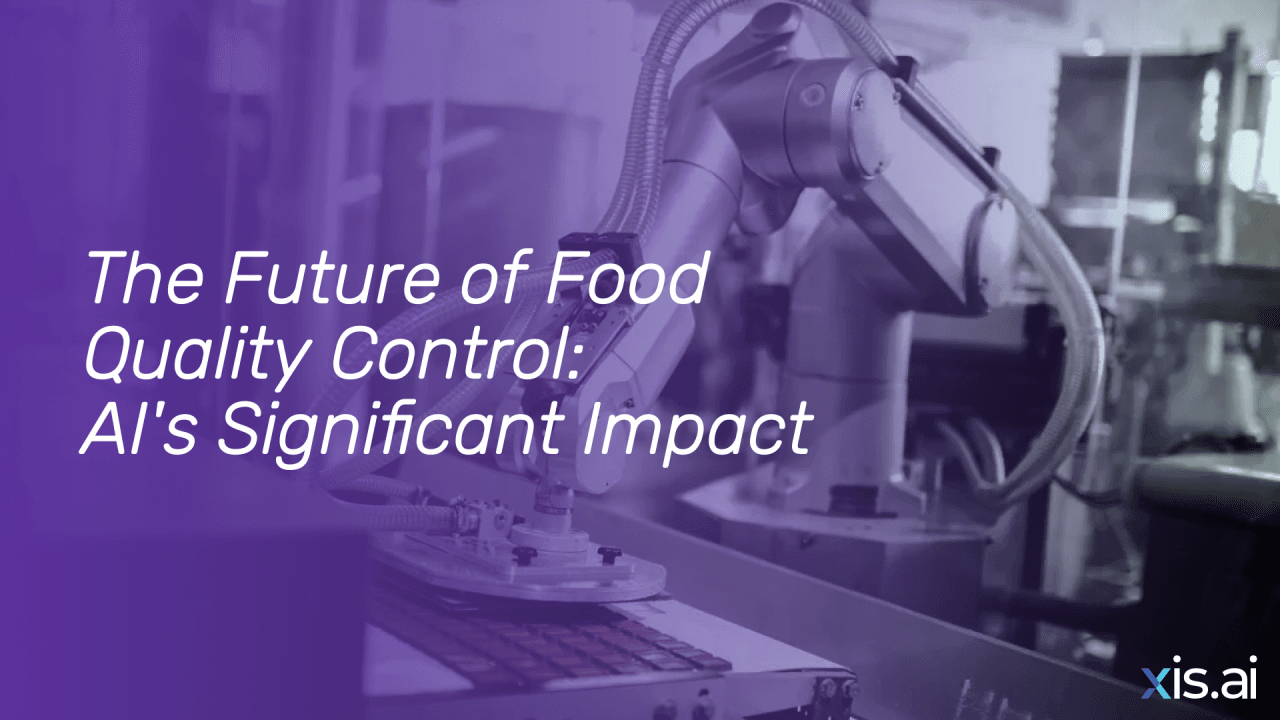
Introduction
The food industry has long struggled with quality control challenges, such as contamination, spoilage, and mislabeling, all of which pose serious risks to consumer health. Traditional inspection methods, relying on human checks and manual testing, are often slow, labor-intensive, and prone to errors. With growing pressure to meet strict quality, safety, and regulatory standards, these outdated approaches are no longer sufficient. Thankfully, Artificial Intelligence (AI) is stepping in to transform quality inspections. By utilizing advanced technologies like computer vision and machine learning, AI offers a faster, more accurate, and reliable way to inspect food products, ultimately leading to improved safety and compliance across the industry.
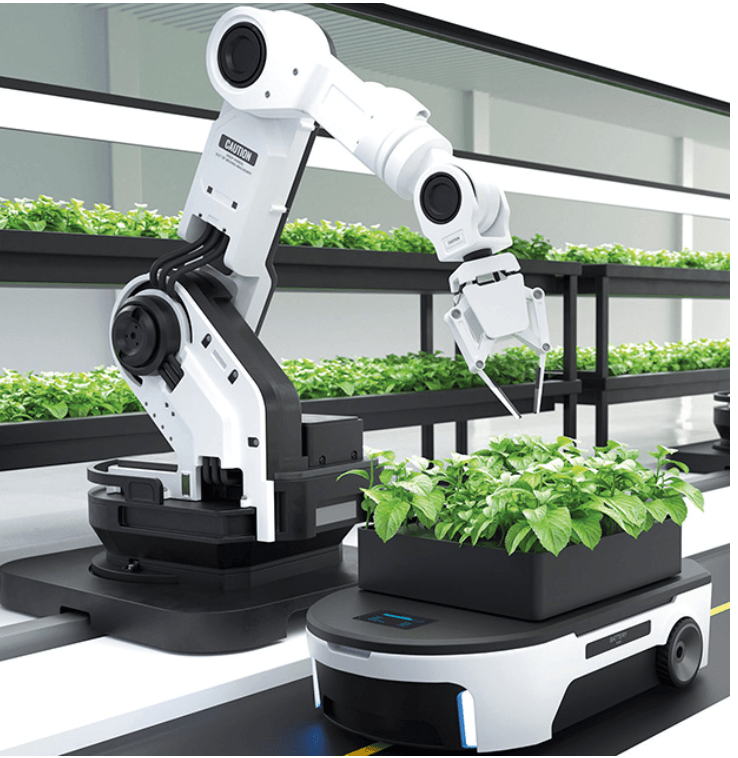
Key Benefits
1. Improved Quality Assurance
AI utilizes computer vision technology to automate the inspection process, identifying defects with remarkable accuracy and minimizing human mistakes.
2. Predictive Analytics
Machine learning algorithms analyze production data to identify patterns and anticipate potential quality issues before they arise.
3. Real-Time Monitoring
AI-powered sensors and cameras continuously monitor production lines, providing instant feedback and enabling immediate corrective actions.
4. Enhanced Traceability
The combination of AI and blockchain technology boosts traceability from the farm to the consumer, establishing clear and transparent records of the entire production process.
5. Reducing Costs
By automating quality inspections, manufacturers can cut down on labor expenses, decrease product waste, and achieve substantial savings.
6. Compliance with Regulations
AI simplifies documentation and reporting, aiding in adherence to food safety standards and reducing the risks linked to non-compliance.
7. Automated Defect Detection
AI algorithms can be developed to identify particular defects, allowing for the automatic rejection of faulty products.
8. Enhanced Food Safety
AI helps detect potential food safety hazards, such as microbial contamination or allergens, ensuring products meet regulatory requirements.
9. Improved Efficiency and Productivity
By automating inspection tasks, AI significantly reduces labor costs and increases overall productivity.
10. Real-Time Insights
AI systems provide insights into production processes, enabling process optimization and informed decision-making.
11. Insightful Analysis
AI analyzes inspection data to identify trends and potential root causes of quality issues, facilitating data-driven decision-making.
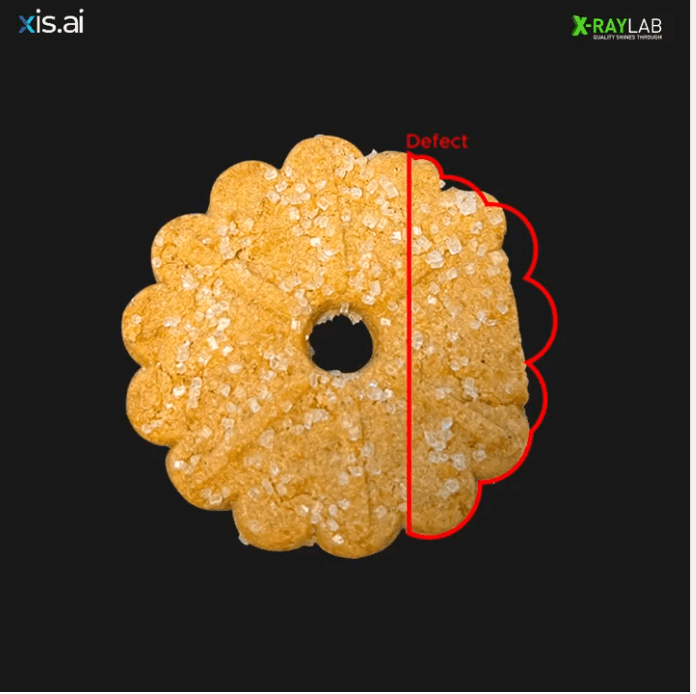
Techniques of AI-Powered Quality Inspection in the Food Industry
Quality inspection in the food industry, enhanced by AI, utilizes a range of methods, with a strong focus on computer vision and machine learning.
Below are some of the main techniques used:
1. Computer Vision Techniques
- Image Acquisition: High-resolution cameras and sensors are used to capture images or videos of food products.
- Image Preprocessing: Preprocessing techniques are applied to enhance image quality, remove noise, and normalize lighting conditions for consistent analysis.
- Feature Extraction: AI algorithms extract relevant features from the images, such as color, texture, shape, and patterns, which are critical for identifying defects and ensuring product quality.
- Object Detection and Localization: Algorithms identify and locate objects of interest, such as defects, contaminants, or specific food items, within the images.
- Image Segmentation: Images are divided into regions or segments corresponding to different objects or features, enabling precise analysis of specific areas.
- Object Classification: Detected objects are classified into predefined categories, such as “defective” or “non-defective,” ensuring only high-quality products proceed in the production line.
2. Machine Learning Techniques:
Supervised Learning: Models are trained on labeled datasets, where each image is associated with a correct class or label.
Common algorithms include:
- Convolutional Neural Networks (CNNs): Highly effective for image recognition and classification tasks in food quality inspection.
- Support Vector Machines (SVMs): Used for classification and regression tasks, identifying patterns associated with defects.
- Random Forest: An ensemble learning method that combines multiple decision trees, improving accuracy in defect detection.
Unsupervised Learning: Models learn patterns and structures in data without explicit labels.
Techniques include:
- Clustering: Groups similar data points together to identify potential defects.
- Dimensionality Reduction: Reduces the number of features while preserving important information, aiding in more efficient analysis.
3. Transfer Learning
Pre-trained models are fine-tuned on smaller, specific datasets, accelerating the training process and improving performance on food inspection tasks.
4. Reinforcement Learning
Models are trained to make decisions based on rewards and punishments, optimizing inspection strategies over time.
5. Deep Learning Techniques
Deep Convolutional Neural Networks (DCNNs): Complex neural networks with multiple layers capable of learning intricate patterns and features, making them particularly effective in visual inspection tasks.
Generative Adversarial Networks (GANs): Generate synthetic images for data augmentation and anomaly detection, improving the robustness of inspection models.
6. Hybrid Approaches
Ensemble Methods: Combine different AI models to improve the accuracy and dependability of defect detection and classification.
Multi-sensor Fusion: Integrates data from different sensors (e.g., cameras, infrared, hyperspectral) for more comprehensive analysis, enhancing the reliability of quality assessments.
Additional Techniques
- Predictive Analytics: Utilizes historical data to forecast future quality issues. By analyzing trends and patterns, manufacturers can proactively reduce defects and ensure consistent product quality.
- Sensor Technology: AI-powered sensors monitor environmental factors such as temperature, humidity, and gas levels during food production. These sensors help detect conditions that may lead to spoilage or contamination, enabling timely interventions.
- Data Fusion: Combines information from multiple sources, such as sensors, cameras, and production data, to provide a comprehensive view of quality metrics. The comprehensive method improves decision-making by providing a more profound understanding of the production process.
- Natural Language Processing (NLP): Analyzes customer feedback, reviews, and social media mentions to identify quality-related issues. This helps manufacturers understand consumer preferences and concerns, allowing them to improve product quality.
- Blockchain Integration: Enhances traceability and transparency in the food supply chain. AI can analyze blockchain data to identify potential quality issues and ensure compliance with safety regulations.
- Anomaly Detection: Uses statistical methods and machine learning algorithms to identify unusual patterns in production data, alerting manufacturers to potential quality issues before they escalate.
- Automated Feedback Systems: AI systems provide real-time feedback to operators based on quality inspection results, enabling immediate corrective actions and adjustments to the production process, ensuring that quality standards are consistently met.
By combining these approaches, AI-powered quality inspection systems deliver accurate, efficient, and reliable solutions for the food industry, ensuring product safety and meeting consumer demands.
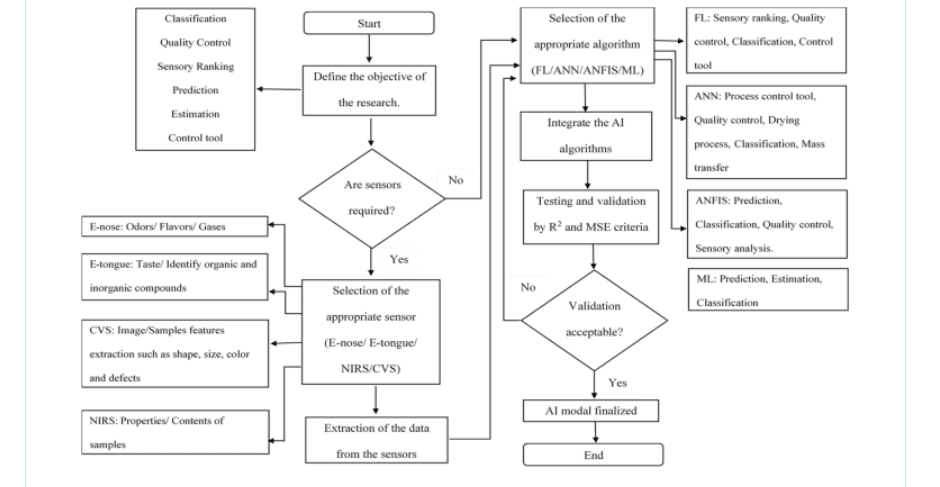
Applications of AI-Powered Quality Inspection in the Food Industry
The food industry is transforming thanks to AI-driven quality inspection, which is improving quality control, minimizing defects, and boosting efficiency. Here’s a look at how AI is significantly influencing different phases of food production and supply chain management.
1. Automated Inspection Systems
Real-Time Defect and Contaminant Detection: AI-driven computer vision systems automatically inspect food products, spotting defects like cracks, discoloration, odd shapes, and foreign objects instantly. This helps ensure that only top-quality products are delivered to consumers, reducing the chances of recalls and unhappy customers.
Packaging Inspection: AI inspects packaging for defects, including incorrect labeling, sealing issues, or damaged containers, ensuring product integrity and compliance with labeling regulations.
Packaging Inspection: AI inspects packaging for defects, including incorrect labeling, sealing issues, or damaged containers, ensuring product integrity and compliance with labeling regulations.
2. Quality Control and Assurance
Streamlined Quality Control: AI reduces the need for manual inspections by detecting defects earlier in the production process, leading to significant reductions in defects and improving overall product quality. For example, a global food and beverage company achieved a 20% reduction in defects using AI.
Consistency Monitoring: AI systems analyze attributes such as size, shape, and weight to ensure product uniformity, which is crucial for maintaining brand integrity and customer satisfaction.
Consistency Monitoring: AI systems analyze attributes such as size, shape, and weight to ensure product uniformity, which is crucial for maintaining brand integrity and customer satisfaction.
3. Sensory Analysis:
Texture, Flavor, and Aroma Evaluation: AI-powered systems analyze sensory attributes, such as texture (e.g., meat tenderness, vegetable crispiness), flavor profiles, and aroma. This helps maintain consistency in sensory quality across batches, which is vital for products like sauces, snacks, and beverages.
4. Predictive Maintenance
Equipment Monitoring and Energy Efficiency: AI monitors the performance of food processing equipment, predicting potential failures and enabling preventive maintenance. It also optimizes energy consumption in food production facilities, reducing costs and environmental impact.
5. Food Sorting and Classification
Product Sorting and Classification: AI-powered computer vision systems sort and classify food products based on quality criteria, ensuring the production of high-quality yields. This technology enhances efficiency in food sorting, classification, and prediction, contributing to better product quality.
6. Supply Chain Traceability and Optimization
Product Tracking and Provenance Verification: AI tracks food products throughout the supply chain, ensuring traceability and compliance with food safety regulations. It also verifies the origin and authenticity of products, protecting consumers from counterfeit or substandard goods.
Supply Chain Optimization: AI optimizes various aspects of the supply chain, including crop selection, logistics, and food delivery, leading to more efficient operations and reduced costs.
Supply Chain Optimization: AI optimizes various aspects of the supply chain, including crop selection, logistics, and food delivery, leading to more efficient operations and reduced costs.
7. Food Safety and Allergen Detection
Contaminant Detection: AI detects contaminants such as microbial pathogens, allergens, and chemical residues, enhancing food safety by preventing contaminated products from reaching the market.
Allergen Detection: AI analyzes food samples for the presence of allergens, ensuring products are safe for consumption by individuals with specific dietary restrictions.
Allergen Detection: AI analyzes food samples for the presence of allergens, ensuring products are safe for consumption by individuals with specific dietary restrictions.
8. Ripeness, Freshness, and Spoilage Assessment
Ripeness and Freshness Evaluation: AI-powered image analysis assesses the ripeness and freshness of fruits and vegetables by evaluating color, texture, and shape, allowing for better sorting and categorization.
Spoilage Detection: AI sensors detect early signs of spoilage in perishable goods by monitoring environmental factors like temperature, humidity, and gas emissions. Early detection allows for timely interventions, reducing food waste and ensuring product quality.
Spoilage Detection: AI sensors detect early signs of spoilage in perishable goods by monitoring environmental factors like temperature, humidity, and gas emissions. Early detection allows for timely interventions, reducing food waste and ensuring product quality.
9. Shelf-Life Prediction and Waste Reduction
Predictive Analytics: AI models use historical data and current conditions to estimate product shelf life, helping manufacturers optimize inventory management and reduce waste by ensuring products are used or sold before expiration.
Waste Reduction: By accurately predicting product quality and shelf life, AI-powered systems help minimize food waste, leading to more sustainable production practices and cost savings.
Waste Reduction: By accurately predicting product quality and shelf life, AI-powered systems help minimize food waste, leading to more sustainable production practices and cost savings.
10. Regulatory Compliance and Customizable Quality Standards
Regulatory Compliance: AI systems help manufacturers comply with food safety regulations by continuously monitoring and documenting inspection results, reducing the risk of non-compliance penalties and facilitating efficient product recalls if necessary.
Customizable Quality Standards: AI allows manufacturers to set and adjust quality standards based on specific customer requirements or market demands, providing flexibility in product offerings and meeting diverse consumer expectations.
These applications illustrate that AI-powered quality inspection enhances efficiency, safety, and sustainability in the food industry, ultimately benefiting both producers and consumers by ensuring high-quality, safe, and consistent food products.
Customizable Quality Standards: AI allows manufacturers to set and adjust quality standards based on specific customer requirements or market demands, providing flexibility in product offerings and meeting diverse consumer expectations.
These applications illustrate that AI-powered quality inspection enhances efficiency, safety, and sustainability in the food industry, ultimately benefiting both producers and consumers by ensuring high-quality, safe, and consistent food products.
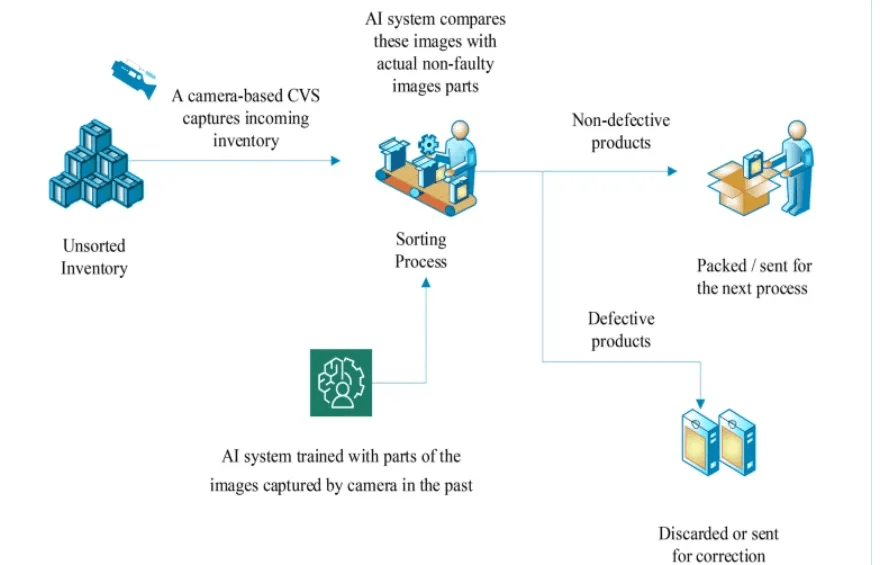
Examples of AI Applications in the Food Industry
- Meat Inspection: AI can automatically inspect meat products for defects, contaminants, and foreign objects.
- Seafood Inspection: AI can detect parasites, contaminants, and quality issues in seafood products.
- Fruit and Vegetable Grading: AI can accurately grade fruits and vegetables based on factors such as size, color, and quality.
- Dairy Product Inspection: AI can monitor the quality of dairy products, ensuring consistency and safety.
Food manufacturers can enhance product quality, boost food safety, lower expenses, and satisfy the growing expectations of consumers and regulatory agencies by utilizing AI-driven quality inspection.
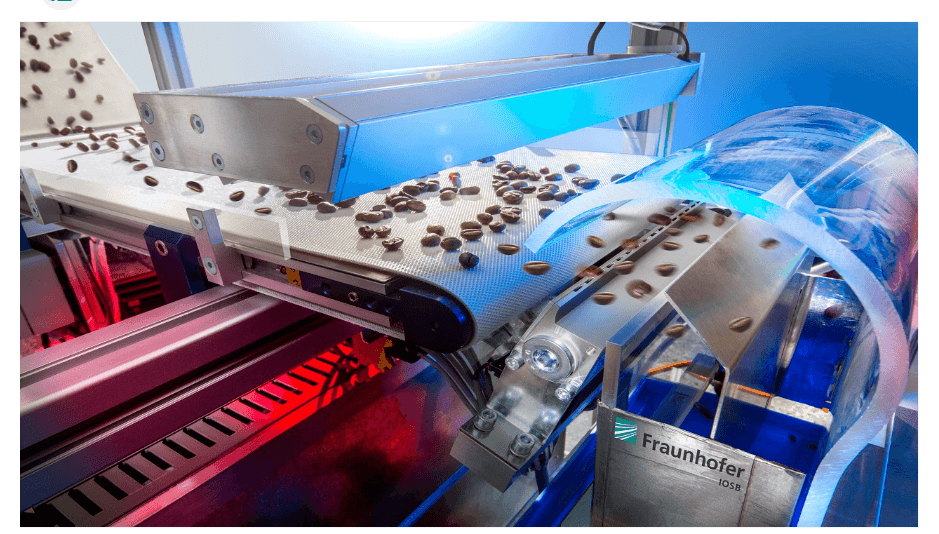
Challenges of AI-Powered Quality Inspection in the Food Industry
Adopting AI-driven quality inspection in the food industry presents several challenges. High-quality, labeled datasets are crucial for accurate AI analysis, but data variability and privacy concerns complicate deployment. The significant initial investment and ongoing maintenance costs can be burdensome, especially for smaller businesses. The complexity of food products and the difficulty of integrating AI into existing workflows further add to the challenges. Ethical concerns, including job displacement and maintaining consumer trust, also require attention. Despite these hurdles, AI-driven systems offer enhanced accuracy, efficiency, and reduced human error, making it essential to address these challenges for successful implementation.
The Future of AI in Food Quality Inspection
As artificial intelligence technology progresses, we can anticipate a surge of innovative uses within the food sector. For instance, robots powered by AI could take on roles like sorting, packaging, and inspecting, which would further streamline the production process. Moreover, combining AI with other technologies, such as blockchain and the Internet of Things (IoT), could enhance transparency and traceability throughout the food supply chain. Ultimately, AI is transforming quality inspection in the food industry by delivering improved accuracy, efficiency, and consistency. Utilizing the power of AI, food manufacturers can ensure the safety of their products, enhance quality, and meet the growing demands of consumers and regulatory bodies.
Conclusion
Artificial intelligence is transforming the food industry with innovative quality inspection solutions using computer vision, machine learning, and deep learning. These AI-powered systems enhance accuracy, efficiency, and consistency through automated defect detection, real-time monitoring, predictive maintenance, and supply chain traceability. However, successful adoption requires addressing challenges like data quality, model complexity, integration, and ethical considerations. By investing in research and development and promoting collaboration between humans and AI, the food industry can utilize AI to ensure product safety, improve quality, and meet consumer demands. As AI advances, it will play a crucial role in delivering safe, high-quality food products while reducing waste and operational costs.
About xis.ai
xis.ai automates visual quality inspection with AI and robotics. With a camera and no code computer vision platform that enables non-technical industrial users to develop, deploy, and use automated visual inspection (AOI) in any industry in minutes.
References
https://link.springer.com/article/10.1007/s12393-021-09290-z
https://www.iosb.fraunhofer.de/en/projects-and-products/food-quality-with-food-control-systems-foodcontrol.html
https://www.foodqualityandsafety.com/article/how-artificial-intelligence-is-used-in-the-food-industry/
https://www.iosb.fraunhofer.de/en/projects-and-products/food-quality-with-food-control-systems-foodcontrol.html
https://www.foodqualityandsafety.com/article/how-artificial-intelligence-is-used-in-the-food-industry/
Comment
0Comments
No comments yet.