The Evolution of Non-Destructive Testing Through AI
Recent Post:
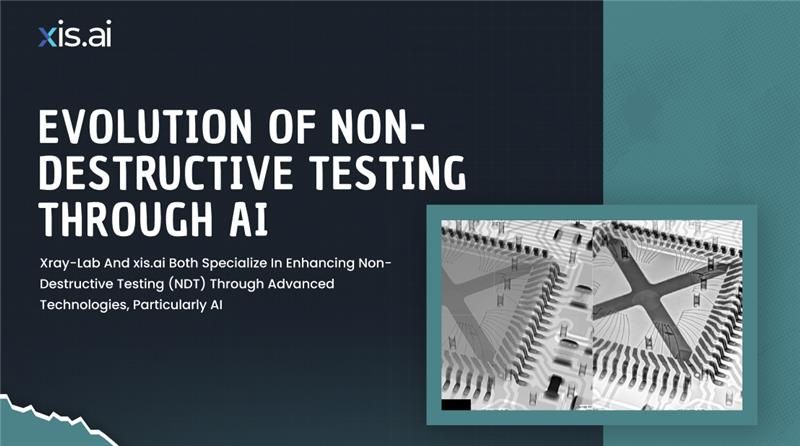
Non-destructive testing (NDT), is a very active process throughout various industries such as aerospace, automotive, construction, and energy, where materials and structures are ensured their integrity and safety without causing any harm. Throughout the years, NDT has transformed in a way, and now, it is leaping thanks to Artificial Intelligence.
Replacing the conventional way of conducting NDT, AI automates data analysis, increases accuracy in defect detection, allows for predictive maintenance usage, and supports change based on relevant insights. NDT 4.0 profited greatly from the use of advanced data analysis, digital twins, more efficient inspection methods, and automatic defect recognition (ADR), where the efficiency, accuracy, and management have been greatly improved transforming NDT techniques about the entire infrastructure from end to end so that several targets such as risk reduction and quality assurance have been successfully met.
The Traditional Landscape of NDT
To this day, manual inspections using rudimentary instruments constitute a large portion of NDT. Tactics such as ultrasonic testing, radiographic testing, and magnetic particle testing call for skilled specialists to conclude. Despite their effectiveness, these approaches were, in general, slow, subject to human mistakes, and not easily expandable. Human personnel also represented a major subjective element This made it difficult to ensure the uniformity of procedures in various types of economy.
Introduction of Artificial Intelligence
By removing many of these limitations, artificial intelligence has altered the NDT process. Parallel to this, however, the data generated is mined, patterns are discovered, and predictive analytics are performed. These tasks are transforming the way inspections are carried out. The synergy of artificial intelligence with imaging and sensing technologies enables various traditional methods of non-destructive testing to be enhanced in terms of accuracy and efficiency, presenting new opportunities for technology.
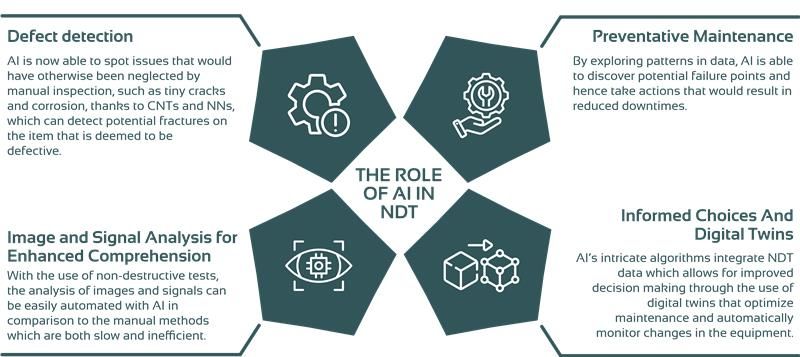
The Role of AI in NDT
Key Points of NDT Procedures Using Artificial Intelligence
- Automated Data Analysis
AI-based systems can harness more data from ultrasonic, radiographic, and thermographic techniques and carry out the analysis within a few seconds. Such amalgamation provides a conducive environment for easily spotting patterns and abnormalities which in turn leads to more rational results.
- Defect Detection Improvement
Training machine learning models, leveraging large databases, enables the simultaneous detection and classification of a higher number of defects. This facilitates greater sensitivity and allows for earlier detection of issues that could have resulted in failure and greater maintenance efficiencies.
- Predictive Maintenance
By spotting patterns within the inspection data trends, AI can ascertain any failures across equipment before they occur. Such predictions allow for corrective maintenance action to be taken and ensure that the time taken to augment efficiency and operational productivity with the elimination of breakdowns is drastically decreased.
- Real-Time Monitoring
AI systems can provide the user with instant feedback when dealing with infrastructure inspections that require breaking down. Such technologies and systems enable corrective adjustments to be quickly ushered in ensuring that the inspection is not only accurate but also shrinking the waiting time.
- Digital Twinning
The phrase “Twinning” is often associated with 4D modeling, however, in NDT 4.0 it encompasses the whole entity and its respective models. NDT 4.0’s key technology is its ability to simulate real-life entities and advise suggested actions based on said model. With the help of a digital twin, one can analyze a virtual model of a physical object and more accurately estimate its future performance.
- Standardization and Objectivity
Human mitigates the inspections, AI eradicates the bias by making use of the same algorithms and criteria all the time. Such eliminations yield similar results across different types of inspections and various nations crucially ensuring reliability.
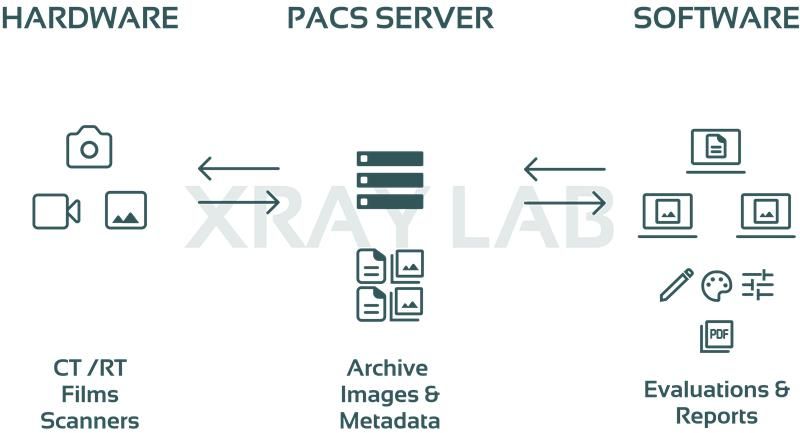
Digital setup with hardware, PACS, and software
Applications of AI in NDT
- Assignment of Inspection and Recognition Automation
With AI, a component can be automatically recognized from an image, and inspection instructions can be issued. The software can overlay a template over an image to show the area where an inspection is needed as well as determine data during an inspection for feeding into the Integrated Data Management System (IDMS).
- Detection of Erosion, Corrosion, and Deposits:
An image-capturing AI tool can identify and locate erosion, corrosion, and deposits from test images with ease, which leads to an increase in accuracy and efficiency. In addition to this, it allows for quicker and more efficient inspections as there is a decreased need for manual review.
- Measurement Automation:
An AI algorithm can identify the thinnest area of a pipe or any other pattern and measure it. This cuts down on human error while at the same time increasing accuracy in the measuring process.
- Covered Item/Image detection (CID)
AI can scan and mark images that need to be analyzed further by looking for any issues, such as screws missed on connecting joints, images not being quality checked, or film errors. This AI technology can be applied to radiographic inspections which are non-picture-based inspections of Ultrasonic data.
- Detail analysis of single items/images(SIDA)
AI capable of visually inspecting items can inspect individual items in depth for defects, take wall thickness measurements, and recognize checking jigs test setups. It can also inspect for film flaws, and recognize text and IQI measurements enhancing inspectors' decision-making capability.
- Image Denoising, Superresolution, and Enhancement
Artificial Intelligence (AI) significantly reduces image noise, enhances the resolution, and improves the quality of images, especially in harsh conditions. For example, AI can eliminate disturbance from weld images and refine defect detection performance which assists human evaluators in identifying those issues more accurately.
- Automatic Defect Recognition (ADR)
AI can precisely detect and classify defects within images thus assisting inspectors to make more accurate and faster identification of issues. It employs supervised learning in the identification of defects using training images with labels and uses image classification, object detection, or instance segmentation algorithms for various stated inspection needs.
Performance of NDT Without AI
Strengths:
- Reliability: Ultrasound, radiography, and dye penetration testing have been used in practice for a long time and have found a wide range of applications in business and in many industries.
- Inspector Qualification: Inspectors put a lot of experience in their work.
- Wide Applications: Such methods are appropriate for a large range of applications including aerospace, oil, and gas industries.
- Compliance: Industry requirements are met.
Limitations:
1. Human Error: These procedures require a lot of skill, increasing the variability and possibility of fatigue-induced errors.
2. Time Intense: Manual operations are laborious on time regarding preparation and analysis.
3. Detection Coverage Gaps: Minor or concealed errors may be overlooked.
4. Device Limitations: Old instrumentations fail to handle outlying defects and noise.
5. Lack of forecasting ability: Concentrates only on existing shortcomings.
Performance Characteristics
1. POD Values:
- The best evaluators get an 85.2 probability of detection for true and true negatives.
- This gets a 2.1% probability of a false alarm on the other side.
2. Binary Decision Dynamics:
- True Positive: The sample that turned out defective is correctly rejected.
- True Negative: A sample that turns out free of defects is permitted.
- False Positive: The sample that turned out free of defects is incorrectly rejected.
- False Negative: A Defective sample is scrutinized and flaws are missed.
3. Coverage and Operational Speed: There is a need for multiple examinations for the large area which takes up more time and increases the operational cost.
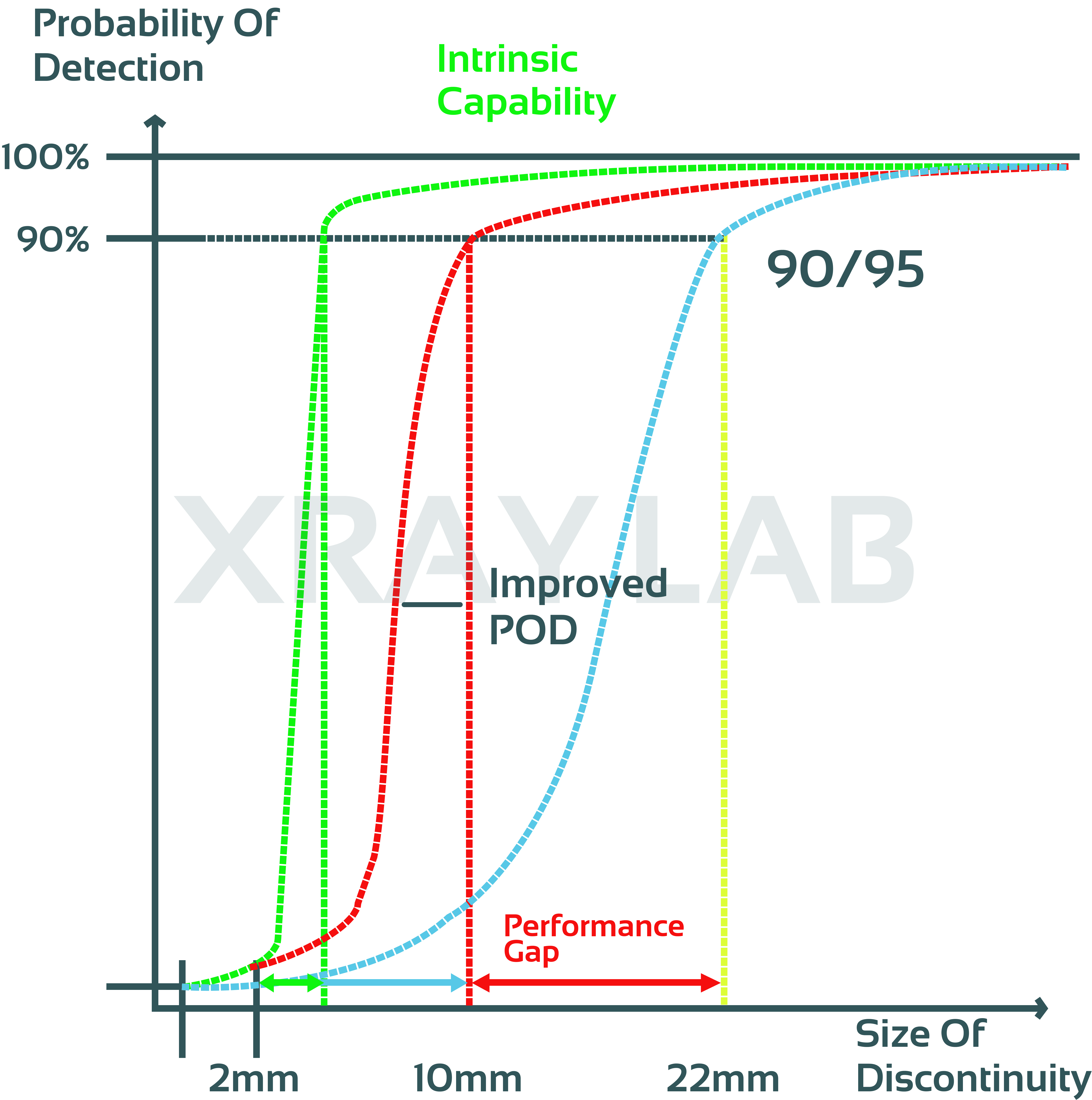
Comparing POD curves to highlight the performance difference
How can Artificial Intelligence improve NDT?
Artificial Intelligence (AI) can complement the human role in Non-Destructive Testing (NDT) by decreasing the bias, increasing the consistency, and automating the processes. This is how it works:
1. Easing the Role of Human Factors:
Automated Defect Detection: The possibility of human inspectors overlooking defects due to fatigue or bias is reduced since AI algorithms are superior at analyzing large amounts of data to find errors.
Standardized Evaluation: There are no different criteria that are applied by different inspectors or during shifts because AI uses the same set.
2. Improving Accuracy and Degree of Consistency:
High Sensitivity: Optimally designed machine learning models can discover flaws that would otherwise be erringly overlooked by most because of the extensive defect data they were trained on.
Image and Signal Processing: AI uses algorithms that improve the inspection data’s signal-to-noise ratio and increase minor details thereby making flaws easy to identify.
3. Real-Time Feedback and Interventions:
Continuous Monitoring: AI sensors that are embedded in components can provide insights in real-time without the reliance or need for a manual review.
On-the-Spot Analysis: Decision-making is enhanced during inspections as AI provides fast ratings cutting the need for long waits.
4. Emphasizing on Objectivity:
Objective Assessments: It is a complicated task that AI does not attempt since it aims to remain data-focused and eschews human error bias.
Pattern Recognition: Individual interpretation and experience-based recognition flaws are eliminated through algorithms.
5. Assisting Predictive Maintenance:
Data-Driven Insights: Artificial intelligence utilizes past and current data to forecast any possible failures and assists in planning for maintenance before a problem occurs.
Proactive Decision Making: AI helps to prevent defects from occurring hence eliminating the need for a human response which is prone to more errors.
6. Optimizing and simplifying Workflows:
Automated Reporting: With AI systems, independent and detailed reports are always provided which enables a human inspector to carry out intricate routines.
Batch Processing: The AI can evaluate some sets of data or images at the same time increasing the rate of inspection as well as decreasing the workload.
By integrating AI, NDT can:
- Ensured reliability and consistency of NDT services.
- Increased safety and more time saved as idle time is reduced.
- Decreased costs from manual blunders or unnecessary duplicate inspections.
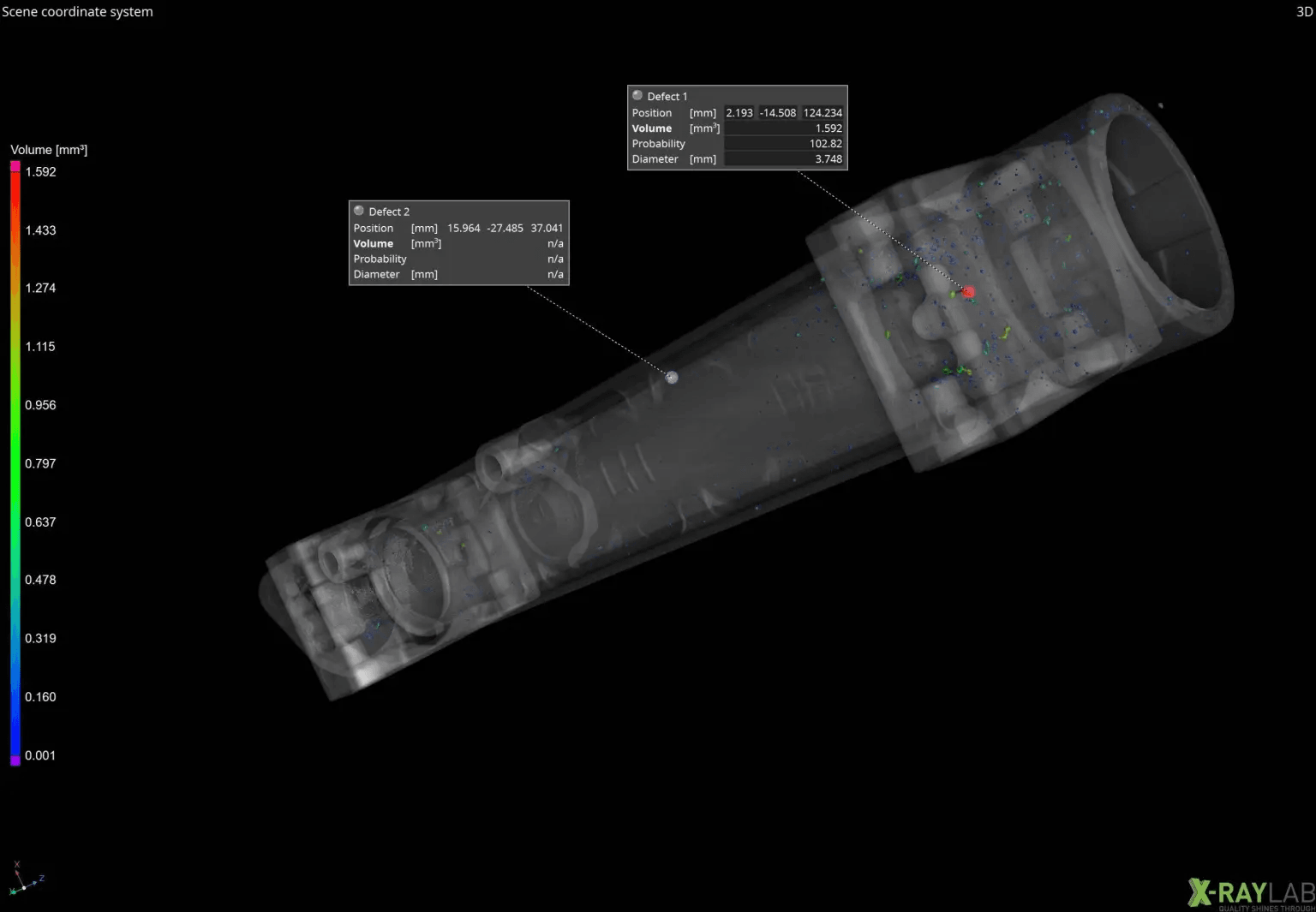
Examples of NDT With the Role of AI:
Few examples of how AI has been applied in NDT scenarios to reduce human factors and improve outcomes:
1. Radiographic Testing (RT) For The Inspection Of Pipelines
Application: AI has algorithms capable of analyzing X-ray images of pipelines and identifying cracks, corrosion, and other structural damage.
Role of AI:
The machine-determined algorithms were fed with considerable data sets and are more accurate in finding defects than the review of humans.
Concern areas are marked by the automated systems for future examination so that the number of false negatives is less than before.
Impact: More defects will be detected, downtime will be decreased, and safety during oil and gas operations will be improved.
2. Ultrasonic Testing (UT) In The Maintenance Of Aircraft
Application: AI-powered ultrasonic systems are employed in finding fatigue corrosion cracks on aircraft parts.
Role of AI:
Anomaly detection is performed through analysis of the ultrasonic waveforms by several AI models.
Now decision-making in real time has less dependence on individuals interpreting complex wave patterns.
Impact: There has been enhanced accuracy and reliability about airworthiness where the time taken during inspection has been greatly reduced.
3. Visual Testing (VT) In Manufacturing Sectors
Application: AI-based machine vision systems check surface defects of automotive parts during their production.
Role of AI:
Images or videos taken are interpreted by deep learning algorithms to find scratches, dents, or misalignment of components.
Systems can understand new defect types through induction-based learning.
Impact: Quality assurance is improved, human fatigue is minimized and rejection during production time is reduced.
4. Infrared Thermography (IRT) in Building Inspections
Application: AI interprets thermal imaging to find insulation failures, moisture damage, or weakness in the architectural framework of buildings.
AI’s Role:
The algorithms embedded in AI clinical assistance devices analyze thermal imaging data and identify hotspots and abnormalities.
Automated systems prepare reports with actionable intelligence.
Impact: Reduced errors in the interpretation of thermal imaging, hence improvement in the reliability of the results, and the completion of inspections at an accelerated rate.
5. Magnetic Particle Testing (MPT) in Railway Maintenance in Railway Maintenance
Application: Systems based on AI analyze the patterns of the magnetic fields to locate discontinuities in railway tracks.
AI's Role:
The deployed machine learning algorithms classify minor differentiations from major defects.
Automated systems perform wide-area inspections without human intervention.
Impact: As AI ensures the identification of critical situations, the risks of trains being thrown off balance are reduced, hence increasing safety.
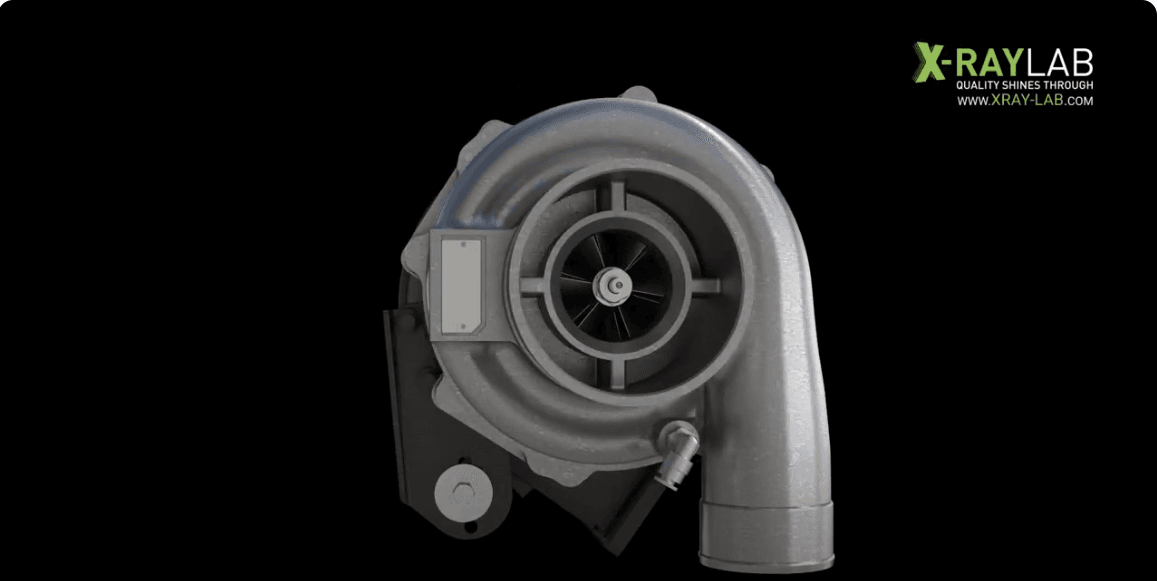
The Outlook on Artificial Intelligence As it Concerns Nondestructive Testing
The development of Nondestructive Testing can be attributed to the implementation of some of the newer technologies that are coming up. Computer vision, deep learning, and IoT systems improvement would enable the construction of more advanced inspection systems. Drones with AI-based sensors, for example, could change the landscape of infrastructural inspections. In the same line, digital twins which are virtual copies of physical assets will allow checking the status of the assets in real-time.
Also, with the availability of AI tools, NDT will be open to less developed industries, thus promoting several inventions in various industries. Artificial intelligence is growing in influence and is likely to impact the industry dissimilarly about NDT in times to come.
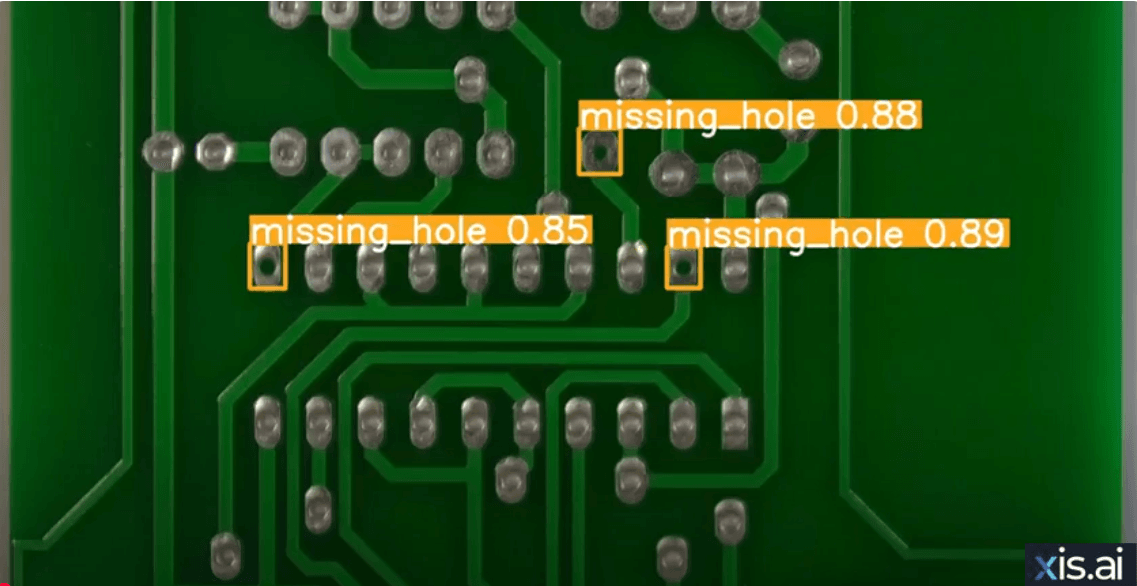
What does Xray-Lab & xis.ai do with AI in NDT?
Xray-Lab and xis.ai both specialize in enhancing Non-Destructive Testing (NDT) through advanced technologies, particularly AI, to improve defect detection and material analysis across various industries. Xray-Lab offers a comprehensive range of NDT methods such as Computed Tomography (CT), X-ray inspection, Ultrasonic Testing (UT), Eddy Current Testing (ET), Dye Penetration Testing (PT), and Endoscopy/Video-scope. With state-of-the-art facilities and AI-based evaluation tools, they ensure precise defect detection and material analysis without damaging products. They use AI to improve NDT by increasing productivity, accuracy, and inspection speed, and emphasize the importance of structured digital databases like PACS to train AI models for better inspection processes.
Similarly, Xis.ai automates visual quality inspections using AI and robotics, focusing on real-time defect detection. Their no-code computer vision platform integrates high-resolution cameras and AI algorithms to identify surface and internal defects, as well as labeling errors. Xis.ai’s solutions simplify inspection workflows, reduce the need for manual inspections, and ensure consistent quality control. Both companies emphasize the transformative potential of AI in NDT, driving innovation, improving efficiency, and maintaining high-quality standards in industries such as manufacturing, pharmaceuticals, aerospace, and automotive.
Comment
0Comments
No comments yet.