AI in Visual Inspection: Tackling Key Challenges
Recent Post:
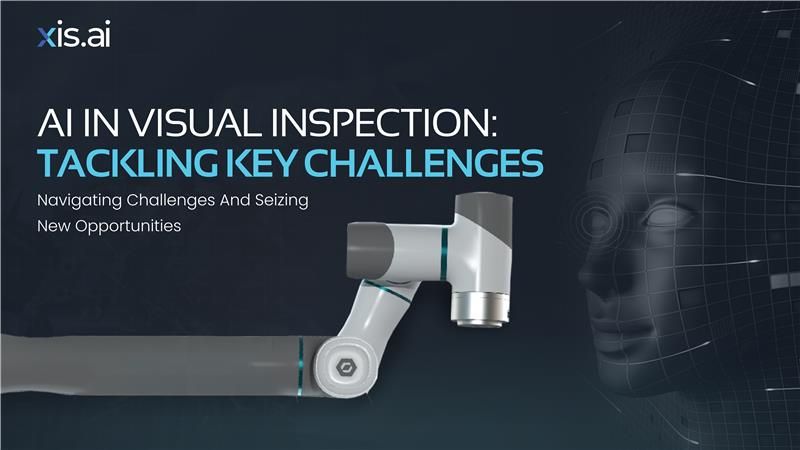
AI visual inspection improves quality control with superior precision, efficiency, and cost savings.
AI systems identify defects with exceptional accuracy and efficiency, augmenting the work done by human inspectors.
Incorporating deep learning and machine learning, AI visual inspection systems can automatically perform defect detection and assembly verification by efficiently processing and interpreting massive quantities of visual data. Unlike manual inspections, AI-powered systems are capable of reliably identifying anomalies in visual data which provides great value to businesses that strive to improve the quality control processes.
How Does AI Visual Inspection Work?
AI systems that inspect visuals make use of technology such as computer vision and machine learning to automate the process of defect detection and quality control within manufacturing and its associated disciplines. Following is the explanation of the workings of these systems in detail.
- Image Capture: Cameras or sensors with great resolution have to be placed at different locations within the production line and these cameras are supposed to capture pictures of the products at crucial phases of the production cycle in detail.
- Data Labeling and Processing: The next step involves labeling and processing these images to prepare datasets for the machine learning models. In this step, the labeled data also helps in teaching the models how to identify multiple patterns, errors, and anomalies within the products.
- Machine Learning Model Training: The subsequent step is training the machine learning model. The algorithms of the machine learning models are fed huge quantities of labeled data so that they can learn how to identify very vague errors or imperfections that would be too tough for a human inspector to identify. During this training process, the system learns to distinguish between defective components and those that are acceptable.
- Real-Time Inference: After the models are trained, they are set on real-time inferencing. As the images for the production process are taken, the AI system reviews them in real time to identify any defects or irregularities.
- Identifying Defects: AI visual inspection systems are capable of detecting issues that range from surface defects, such as cracks, and discoloration to complex assembly verification tasks which might involve missing components and/or misaligned parts.
- Continual Quality Control: AI systems, in contrast to manual inspection, are capable of processing images non-stop. Because of this, they can provide real-time analysis without any interruptions, which equals high throughput and consistent high-quality inspection without delays.
- Automated Reporting and Feedback: When defects are found, the items are flagged as defective, and feedback is automatically sent to the production line. In addition, as a means of helping operators make data-driven decisions to improve process outcomes, detailed reports are also generated.
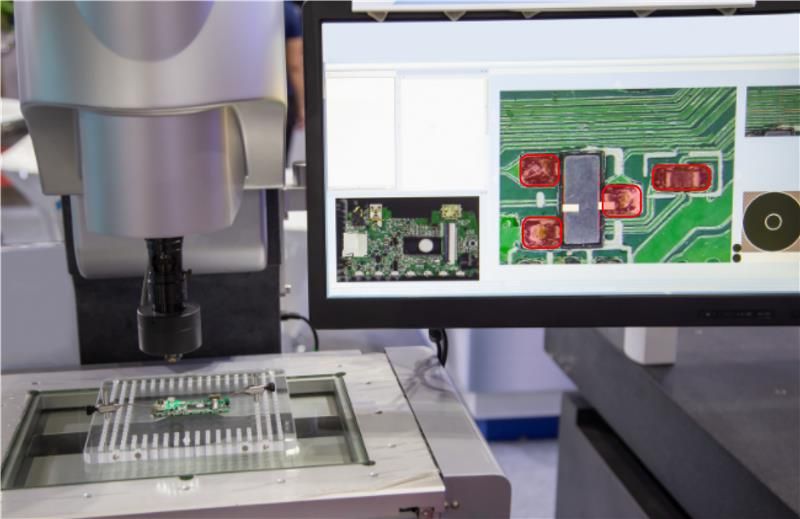
Benefits of AI Visual Inspection
AI visual inspection comes with a myriad of advantages that can drastically enhance an organization’s quality control processes, making it an invaluable asset for industries that demand precision, efficiency, and cost-effectiveness.
Some salient benefits include:
1. Unmatched Accuracy in Defect Detection:
Visual inspection aided by AI is far more accurate and considerate of the defects within a product than manual inspections. These systems are capable of detecting even the minutest being overlooked during manual inspections, guaranteeing an AI Visual Inspection system’s operative requirements.
2. Augmented Effectiveness:
AI-powered visual inspection surpasses traditional approaches in looking at, receiving, and examining large amounts of visual information. Companies can complete work much more quickly than before which allows for increased production rates and the elimination of bottlenecks which are caused by limitations set by people.
3. Expense Reduction:
AI visual inspection saves valuable time and money with every product that requires revision by the inspection system, owing to the limit on rework that it does and avoiding costly recalls. Savings can also be realized through lowered direct human labor with the minimization of human errors and spending, especially within large-scale production.
4. Innovation Through Industry Overlap:
AI-powered visual inspection systems can be deployed across a variety of industries such as manufacturing, automotive, electronics, food, and pharmaceutical industries among others. This multifaceted adoption fosters growth and advancement in many industries allowing the adoption of AI-powered quality assurance in any sector of business.
5. Exploitable Loopholes for Quality Advancement:
AI visual inspection systems produce tremendous amounts of intel that can be studied for patterns and trends. By making sense of this, organizations can uncover the source of defects, streamline their production cycle, and strategize to advance the quality of the output.
6. Business Practices and Legal Risk Supervision:
AI visual inspection systems safeguard compliance with safety and quality regulations avoiding risks such as non-compliance with safety protocols for the highly regulated medical, food, and healthcare industries. In doing so, it facilitates maintaining necessary business certifications while staying free from legal trouble.
7. Reduced Human Error and Bias:
Human inspection has its faults, as an inspector can simply be fatigued, distracted, or complete the inspection with a strong bias, hence rendering the inspection useless. AI Systems crush these risks, assuring the defined inspection accuracy and physical inspection results without deviation.
8. 24/7 Operational Ability:
As much as possible through automation, Artificial Intelligence has improved productivity by controlling production quality. AI systems can function continuously without the need for breaks which guarantees consistent quality evaluation on production lines. The ability of AI to manage business processes enables fulfillment of all operational needs even in the case of insufficient personnel or periods of inactivity.
Key Challenges in AI Visual Inspection:
The challenges that come along with AI inspection systems include the following:
1. Initial Setup and Implementation Costs: Building Artificial Intelligence systems for visual inspections of systems as infrastructure will require the company to invest large sums of capital in equipment, software, and model training.
2. Need for Large and High-Quality Datasets: To train an AI model, large labeled datasets used to be needed but with the development of new vision models, it is now achievable to fine-tune them by only a couple of images which greatly decreases the effort and time needed.
3. Integration with Existing Systems: There is much complexity and customization required for the AI systems to achieve compatibility with older production lines and IT systems.
4. Continuous Monitoring and Maintenance: AI systems need to be constantly supervised by updating models with new information and solving technical problems to ensure optimal status.
5. Data Quality and Quantity: To be adequately trained, AI systems require large, quality data. Insufficient amounts of data can distort or bias the data which results in inaccuracies.
6. Variability in Defects: Variations regarding size, shape, or appearance of manufacturing defects will be extremely difficult for AI to detect without the data set being too nuanced, which poses a problem.
7. Complex Environmental Conditions: False positives or missed defects can be the result of environmental elements such as lighting, shadows, reflection, and camera angles affecting picture clarity.
8. Real-time Accuracy Demand: Just like high-end automotive machinery and semiconductor manufacturing, is expected to be performed instantly while also maintaining accuracy and precision.
9. Need for Scaling AI Models: New product designs and different defect types will require AI models to be retrained, thereby creating challenges for scaling as well as adaptability.
10. Retraining for the New Normal: AI models designed to specifically target certain products or defects typically have a hard time with new or modified blocks, which results in having to re-adjust or retrain them.
11. Reaching an Accuracy Level: Implementing real-time inspection IoT devices means AI models will need to be optimized to reach the desired level of accuracy and speed without draining too many resources.
12. SME Spending Concerning AI Technology: Smaller and medium enterprises will find it extremely hard to afford the upfront one-time expense that comes with purchasing the hardware, software, and training the models.
13. Decision-Making Defects: AI wrongly identifying defects or a lack of systematic defect detection will lead to false rejection or absence of misclassifying.
14. Dilemma of AI Tech: Many industries are still stuck with legacy systems making it extremely hard to apply the complex technologies of AI visual inspection without any customization.
15. Why Certain Defects Were Flagged Or Dismissed: Digital systems that utilize AI technology provide little to no reasoning for defects that were flagged, making tracing the reasoning behind such decisions and understanding the logic behind them a challenge.
How AI Handles Complex Challenges in Visual Inspection:
AI tackles the complications of visual inspection uniquely and effectively:
1. Decreased Initial Costs with Setup and Execution:
- Pre-Trained Models and Turnkey Solutions: Rather than developing AI systems from scratch, businesses can utilize pre-trained models or turnkey solutions that provide integration tools along with a library of pre-built algorithms. This significantly lowers the costs associated with the initial setup.
- Cloud-Based Solutions: Cloud-driven platforms significantly decrease the local infrastructure that is needed, thus allowing businesses to expand their systems without hefty investments.
2. Data Quality and Quantity Management:
- Transfer Learning: AI models can be custom-trained over smaller datasets through transfer learning. This strategy enables the AI to reduce the number of millions of labeled data that are needed by learning from large sets of data from comparable industries.
- Synthetic Data Generation: AI systems could also rely on expanded datasets formed with data techniques such as data augmentation or generative models to increase the robustness of the model.
3. Integration with Existing Systems:
- Modular AI Architectures: Several AI offerings include the use of modular systems and API tools that enable the integration to be done without any hassle. Doing this allows businesses to link their legacy systems to brand-new AI systems without completely changing their infrastructure.
- Edge AI: There is the capability of operating AI models on edge devices which allows applications to process data and make decisions locally in real time which alleviates reliance on bulk central systems. In turn, this minimizes the complications associated with integration issues.
4. Continuous Monitoring and Maintenance:
- Automated Updates and Self-Learning: AI models can autonomously adapt to changes through AI model updates based on real-time data streams. Furthermore, ensuring the self-learning capability is adopted allows for adaptation to changes in manufacturing processes and destructive patterns.
- Cloud Monitoring: Cloud-based AI systems can be monitored in a remote manner which guarantees uninterrupted monitoring, updating, error detection, and maintenance while the system is fully operational.
5. Handling Variability in Defects:
- Deep Learning and Convolutional Neural Networks (CNNs): Deep learning algorithms sensitive to overwhelming structures like CNNs are exceptionally good at discerning objects in images irrespective of whether the images have variations in shape, size, or appearance. It is the proficiency of AI systems in tackling complicated defect variations using advanced techniques rather than older frameworks.
- Adaptive Algorithms: AI systems sustain the ability to adapt to new defect patterns and improve their precision as the number of processed images increases.
6. Fast Processing Times:
- Edge AI: Evidence suggests that AI models are successfully run on edge devices without suffering delays, enabling fast real-time processing which is supremely beneficial for the automotive manufacturing industry.
- Optimized Algorithms: AI systems should be designed to achieve the bare minimum level of computational efficiency without compromising accuracy.
The Path Forward:
AI visual inspection technologies are transforming quality assurance through inexplicably accurate, quick, and cost-efficient measures. They serve to defect detection, productivity enhancement, and quality control across myriad industries. Although they have considerable limitations, such as expensiveness and difficulty in system integration, the value AI brings with processing and understanding large amounts of visual information is hard to ignore. Because of improvements like transfer learning and edge computing, these technologies are increasingly easy to implement and flexible. The progression of AI will no doubt further it as one of the primary means of enabling businesses to outperform competitors and ensure high standards in manufacturing.
Comment
0Comments
No comments yet.