AI Visual Inspection is Redefining the Future of Manufacturing
Recent Post:
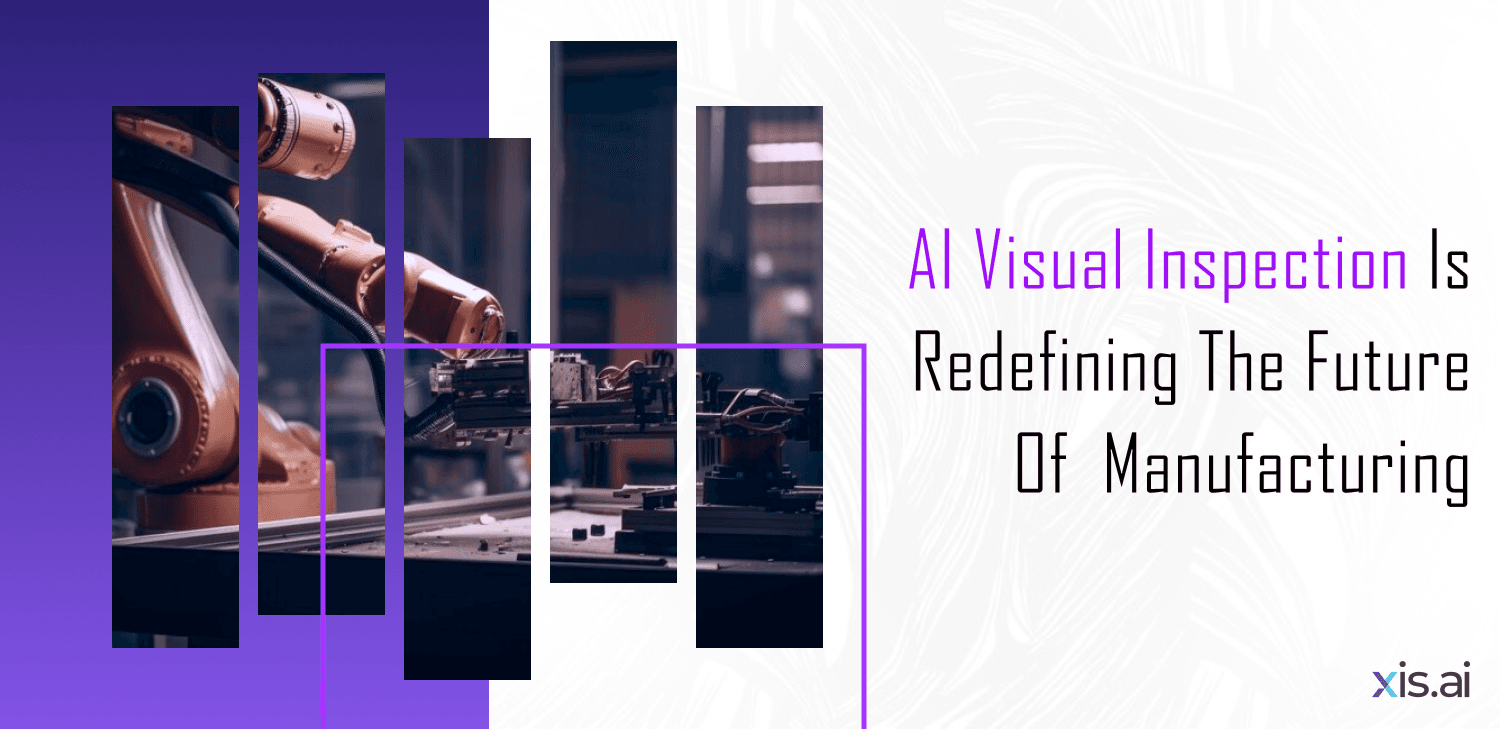
Introduction
In today’s demanding manufacturing industry, even minor mistakes can be expensive. This makes quality control more essential than ever. Traditional inspection methods, which rely heavily on people and are likely to cause errors, find it difficult to keep up with the need for higher-quality products and quicker production.
That’s where AI-powered visual inspection comes in. It’s like having a smart machine that can spot flaws in products instantly. By checking images in real time, these systems can find problems with amazing accuracy, reducing human errors and making sure every product is excellent.
They offer useful insights based on data, improve operational performance, and lower costs by minimizing defects and waste, ultimately helping manufacturers meet industry standards while delivering superior products at scale. For modern manufacturers, AI visual inspection is no longer a luxury but a necessity to maintain a strong position in the market.
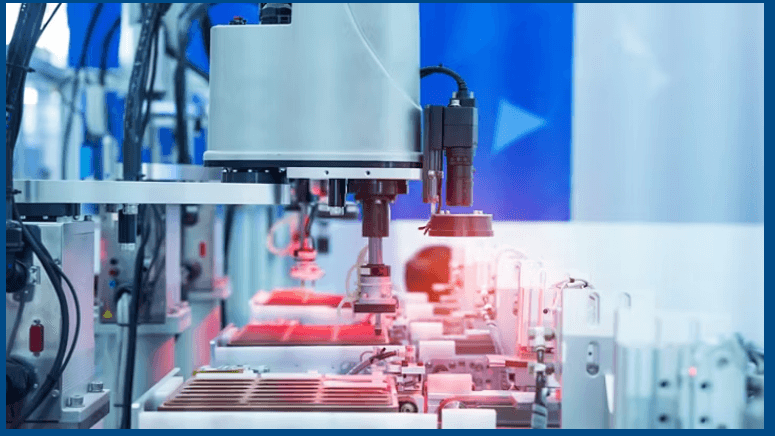
What is Visual Inspection?
Visual inspection is a method used in manufacturing to assess products, components, or materials for defects and ensure they meet established standards. People or automated systems can perform this process, looking for problems like flaws or inconsistencies.
Checking things carefully early in the manufacturing process helps make products better, saves materials, and makes sure they follow all the rules.
In earlier times, inspection people had to do everything by eye. And their speed was not very quick at all, the current conditions lost its real objective. The manufacturer can depend on smooth checks for faster and higher quality products with these intelligent machines.
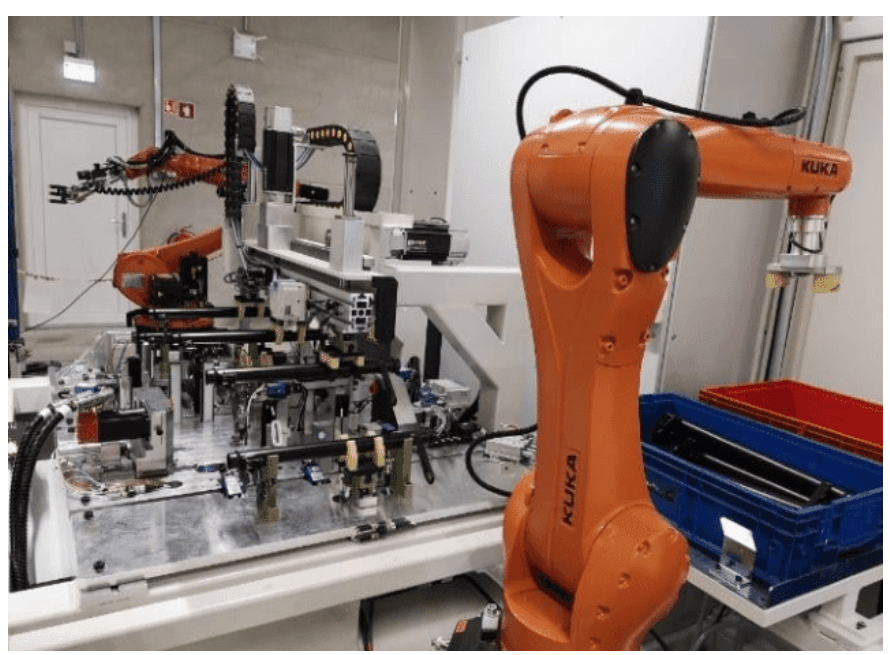
Automated visual inspection system.
The Role of AI Visual Inspection in Manufacturing
For manufacturing, AI visual inspection is now essential. It breaks limitations on the control by changing quality, and into self-defense itself.
With human inspectors in rapid production, the work is not always the same. Advanced technology is used by AI visual inspection systems to look at images and videos of products on the production line, finding defects or problems. Automating the inspection means consistency, speed and accuracy.
This is a digital age technique that gives higher productivity such as previously unobtainable levels of control over quality in production. This technology helps increase productivity and reliability in production, assuring high-quality products. This technology helps increase productivity and reliability in production, assuring high-quality products.
Fundamental Aspects of AI Visual Inspection in Manufacturing
- Machine Learning Algorithms: Machine learning is used by AI systems to improve their ability to detect defects over time. These algorithms have become more effective from previous inspections in identifying issues, adapting to new patterns, and continuously increasing accuracy.
- High-Resolution Imaging: Many AI visual inspection systems use high-resolution cameras to take clear pictures of products. It facilitates more accurate analysis which makes it easy and clear to spot even the smallest defects that could be overlooked by human inspectors.
- Multi-Object Detection: These systems can inspect multiple items at the same time, increasing productivity on production lines. This ability allows manufacturers to process more products in less time, which is important in busy environments.
- Integration with Manufacturing Systems and IoT Devices: AI visual inspection can easily integrate with other manufacturing processes and systems as well as connect with IoT devices. This connection allows for smooth operations and simple data sharing across platforms, improving the overall workflow and demonstrating adaptability to new technology.
- Accessible Interfaces: Many AI visual inspection systems come with straightforward interfaces that simplify monitoring inspections and making necessary adjustments. This easy-to-use design shortens training time and increases overall productivity.
- Individualized Parameters: The inspection rules and settings can be adjusted by users to match their particular quality standards and product needs. This flexibility ensures the inspection process meets the exact requirements of each product.
- Defect Categorization: AI models can categorize defects into different classes, which provides valuable information for root cause analysis and continuous improvement. Manufacturers understand the defects and implement corrective actions with their help.
- Deep Learning: Deep learning techniques are used by AI models to examine large datasets of images which allows them to identify detailed patterns and irregularities that may be hard for human inspectors to detect. This ability greatly improves finding and examining defects.
- Image Evaluation: AI algorithms can analyze different features of images, including color, texture, shape, and size, to identify defects. This detailed evaluation enables a more complete assessment of product quality
- Data Analysis: AI systems can gather and examine inspection data to spot trends and patterns. Based on data, the examination helps in improving production processes, enhancing product quality, and supporting decisions
- Real-Time Inspection: AI systems can analyze images right away providing quick feedback during the inspection. This quick feedback allows for fast changes in production, making sure defects are fixed quickly.
- Safety Protocols: AI visual inspection in manufacturing uses strong safety measures to keep data accurate and private, ensuring the safe handling of sensitive information.
Benefits of AI Visual Inspection in Manufacturing
1. Improved Quality Assurance:
AI visual inspection systems greatly decrease the number of defective products by identifying flaws early in the production process. This results in better quality standards and enhances customer satisfaction. Consequently, manufacturers can establish a strong reputation for quality, which leads to increased customer loyalty and potentially higher sales.
2. Reduced Costs:
Finding defects early reduces the need for rework and scrap, which helps lower overall production costs. By stopping defective products from reaching the market, manufacturers can avoid expenses related to returns, repairs, and losing customer trust. This proactive method can result in significant savings over time.
3. Increased Efficiency:
By automating inspection tasks, AI systems simplify the manufacturing process and decrease the need for manual labor. This improved efficiency allows production lines to work faster, helping manufacturers meet higher demand without sacrificing quality. Automation also lets human workers focus on more complex tasks that need critical thinking.
4. Data Collection and Analysis:
The large amounts of data generated by AI visual inspection systems enable manufacturers to analyze trends and identify recurring issues. This information can inform process optimization strategies and support continuous improvement efforts, helping manufacturers stay competitive in their markets.
5. Real-Time Monitoring:
AI systems provide immediate feedback and alerts during the inspection process, allowing manufacturers to make quick adjustments as needed. This capability helps prevent defects from accumulating and facilitates a more agile production environment where issues can be addressed promptly.
6. Flexibility:
AI visual inspection systems can be easily trained to check many different products and change to new inspection standards without needing a lot of reprogramming. This flexibility makes them ideal for manufacturers with various product lines or those that often launch new products.
7. Reduced Human Fatigue:
By automating inspection tasks, AI systems reduce the reliance on human labor for repetitive and monotonous inspections. This reduction in manual labor minimizes the impact of fatigue and distractions on inspection quality, leading to more reliable outcomes.
8. Enhanced Safety:
In dangerous environments, AI can make workplaces safer by taking over risky inspection tasks, like checking products or heavy machinery. AI visual inspection systems can do these jobs without putting people in danger, helping to create a safer work environment.
9. Speed and Output:
AI visual inspection systems work quickly, allowing them to check more products in less time. This increase in output is important for high-volume manufacturing, helping companies raise production rates while still keeping quality in check.
10. Data-Driven Decision Making:
AI systems produce useful data that can be analyzed to gain insights into production processes. This information helps manufacturers make smart decisions about improving processes, enhancing product designs, and using resources effectively. With real-time data, companies can better respond to changing market needs.
11. Improved Consistency:
AI visual inspection systems provide consistent and reliable results, eliminating the variability often associated with human inspections. This consistency ensures that every product meets the same quality standards, which is crucial for maintaining brand integrity and customer satisfaction.
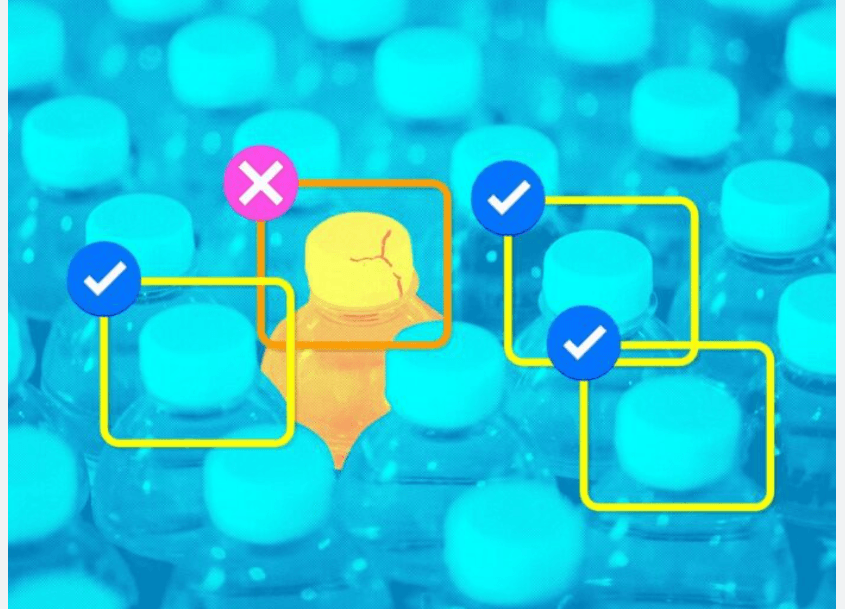
Applications of AI Visual Inspection in Manufacturing
AI visual inspection has emerged as a vital component of contemporary manufacturing, transforming quality control and improving productivity.
Below are some of its main applications:
- Defect Detection: AI improves quality inspection by identifying defects that human inspectors may miss, such as cracks, scratches, or foreign materials. By continuously observing production lines in real time, AI can swiftly detect and rectify issues. This approach promotes product quality, decreases waste, reduces the need for rework, and lowers customer complaints by tackling problems at an early stage.
- Geometric Inspection: The technology provides accurate measurements of dimensions, tolerances, and shapes, ensuring that products adhere to necessary specifications. By refining the inspection process, AI minimizes human error and strengthens production capability. This accuracy in measurement improves product uniformity, leading to dependable and consistent products.
- Assembly Verification: AI provides assurance by ensuring that every component is included and properly assembled. It also detects any misalignments or incorrect placements of parts. This thorough check of assembly improves the product's function and reliability, making sure that the final result works as intended.
- Quality Assurance: For ensuring product compliance by confirming that items meet quality standards and regulations, AI plays an important role. It improves product tracking by monitoring information and history, encouraging accountability. Furthermore, AI-powered quality control reduces customer complaints and enhances consumer trust in the brand.
- Predictive Maintenance: AI helps monitor equipment health by looking at visual data from machines to find signs of wear and tear. It also predicts potential equipment failures, allowing for timely maintenance that reduces downtime. By implementing this, AI helps extend the lifespan of manufacturing equipment, ensuring more potent operations.
- Surface Finish Inspection: Checking surface quality by looking at features like roughness, texture, and appearance, AI can spot flaws such as scratches, pits, or uneven surfaces. It also helps keep products looking good, making sure that they meet visual quality standards.
Implementing AI in Visual Inspection
1. Define Your Goals:
- Identify specific tasks: What do you want the AI to inspect? Defects, measurements, or something else?
- Determine the desired level of accuracy: How precise do the results need to be?
- Consider the environment: Where will the inspection take place? Are there any constraints like lighting conditions or object variations?
2. Gather and Prepare Data
- Gather a large dataset: This should include images or videos of both normal and defective items.
- Label the data: Mark the objects or defects of interest, which can be done manually or with automated tools.
- Prepare the data: Improve the images or videos to make them clearer and more consistent, which might include resizing, cropping, or reducing noise.
3. Select AI Algorithms:
- Think about how complicated the task is: Simple tasks may only need basic image processing methods, while more complex tasks might work better with deep learning techniques like convolutional neural networks (CNNs).
- Test different algorithms: Try out various AI models to see which one fits your needs the best.
- Use transfer learning: If you have a small dataset, consider using a pre-trained model and adjusting it for your task.
4. Training the Model:
- Split the data: Divide your dataset into training, validation, and testing sets.
- Feed the data: Input the training data into the chosen algorithm.
- Optimize parameters: Adjust the model's hyperparameters to improve its performance.
- Monitor progress: Use the validation set to assess the model's accuracy during training.
5. Evaluate, Refine, and Train Users:
- Test the model with the testing set to check how well it works. Look at the results to see where the model has problems and make the needed changes. Keep improving the model until it meets your accuracy goals.
- Train operators and staff on how to use the AI visual inspection system effectively, giving them the skills to monitor it and make any necessary adjustments.
6. Integration with Existing Systems:
- Integrate with existing systems: Connect the AI model to your production environment.
- Monitor performance: Continuously track the model's accuracy and make updates as needed.
- Consider edge computing: For real-time applications, deploy the model on edge devices to reduce latency.
7. Real-Time Processing:
- Monitor the performance of the AI visual inspection system and gather feedback. Regularly update the model with new data and retrain it to improve its accuracy and adapt to changes in the manufacturing process.
8. Compliance and Security:
- Make sure the AI system follows industry rules and standards. Put security measures in place to protect the accuracy and privacy of the data.
Challenges of AI Visual Inspection in Manufacturing
Even with its benefits, AI visual inspection has several challenges:
- Data Quality and Amount: Much high-quality data is needed for AI models to work well. Collecting and labeling this data can take a lot of time and be expensive.
- Lighting Conditions: Changes in lighting can affect how well the AI model works. Keeping the lighting consistent is important for accurate inspections.
- Object Differences: AI can have trouble with objects that change in size, position, or look. Strong algorithms and thorough training data are needed to manage these variations.
- Computational Resources: Advanced AI algorithms, especially deep learning models, need a lot of resources and require powerful hardware to run effectively.
- Combining with Current Systems: Adding AI to current manufacturing processes can be complicated and needs careful planning and coordination.
Limitations of AI Visual Inspection in Manufacturing
- Defect Complexity: Challenges can occur in recognizing defects that are complex and hard to differentiate from normal modifications.
- False Positives and Negatives: Sometimes AI systems can detect defects that are not present there (false positives) or fail to catch actual defects (false negatives).
- Interpretability: It can be difficult to interpret how AI makes decisions, which makes troubleshooting and improving performance harder.
- Cost: The upfront expense for AI visual inspection systems, which includes hardware, software, and data labeling, can be significant.
- Reliance on Training Data: The success of AI depends heavily on the quality and amount of training data provided.
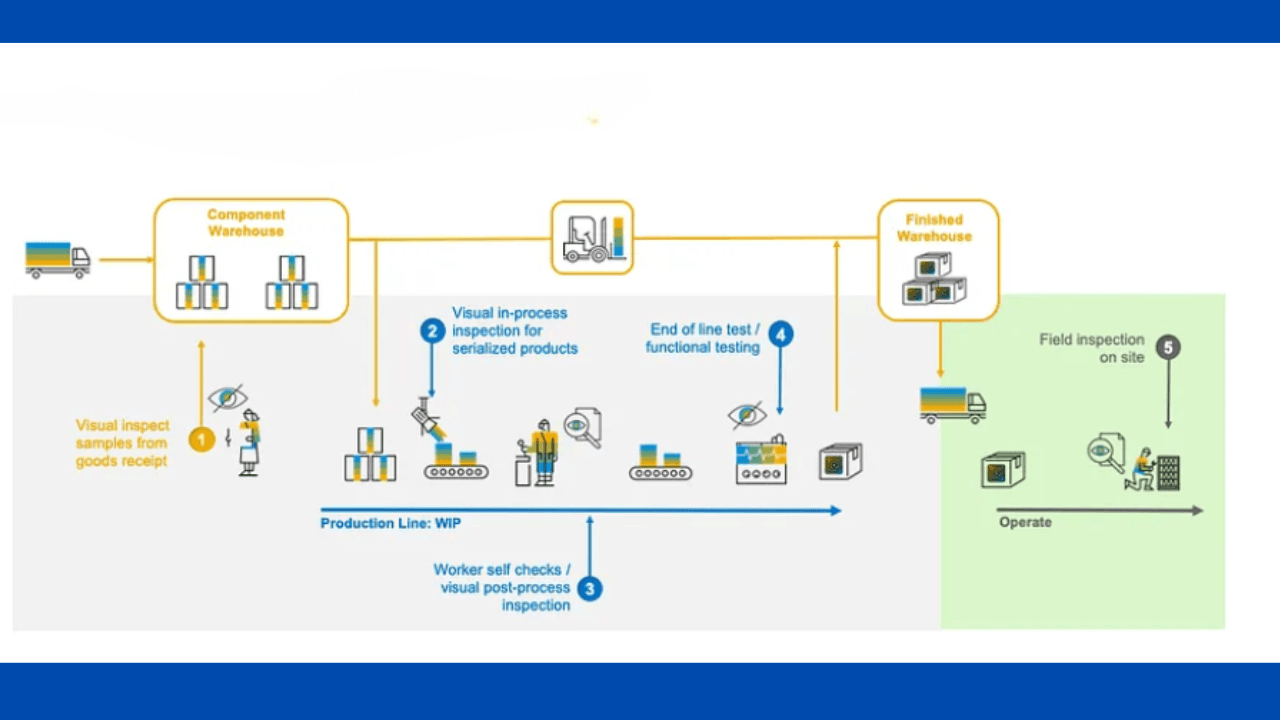
Visual Inspection
Future of AI Visual Inspection in Manufacturing:
The future of AI visual inspection in manufacturing looks vibrant with many key areas ready for improvements. Better algorithms will improve the ability to deal with complex defects and variations while using AI models on local devices will allow for real-time inspections even in remote areas. Data augmentation methods will create synthetic training data, solving data shortages and making models stronger. Additionally, the creation of easy-to-understand AI models will make it simpler to grasp and explain AI decisions. Closer connections with Internet of Things (IoT) devices will allow for real-time data sharing and analysis, further simplifying manufacturing processes. Human help will be less needed for AI systems as automation becomes more innovative leading to better proficiency. In the future AI solutions are expected to offer more customized and adjusted approaches to meet specific manufacturing needs focusing on sustainability by removing waste and improving resource efficiency. Altogether, these innovations will change the manufacturing sector, and will lead to higher quality and operational effectiveness.
Conclusion:
AI visual inspection is changing the manufacturing industry by improving quality control and operational performance. Although there are challenges and limitations, continuous technological advancements are expected to solve these problems and lead to more effective and smart inspection systems. As manufacturers adopt AI solutions, they will be better prepared to meet quality standards and adjust to the changing demands of the market.
About xis.ai:
xis.ai automates visual quality inspection with AI and robotics. With a camera and no code computer vision platform that enables non-technical industrial users to develop, deploy, and use Automated Visual Inspection (AVI) in any industry in minutes.
References:
https://www.intel.com/content/www/us/en/manufacturing/machine-vision.html
https://www.researchgate.net/figure/Automated-visual-inspection-system-8_fig2_333986200
https://www.iotforall.com/ai-visual-inspection-for-defect-detection-in-manufacturing
Comment
0Comments
No comments yet.